WSR-88D Radar Rainfall Estimation: Capabilities, Limitations and Potential Improvements
by
Steven M. Hunter
ABSTRACT
- The WSR-88D Precipitation Processing Subsystem (PPS) represents the first attempt at network-wide operational radar-estimated precipitation in the United States. The system brings a dramatic advancement to operational flood forecasting when compared to earlier radars and rain gage networks. Nevertheless, the system suffers from significant limitations inherent to the use of radar to estimate precipitation. These limitations have persisted despite nearly fifty years of research in the field. Much of the problem has been ascribed to the complex nonlinear relationship between radar reflectivity and rainfall rate at the surface. This relationship has been expressed empirically ("Z-R" power law) and many different ones have resulted from various experiments. Following suit, the WSR-88D software allows a change to the Z-R relationship for differing precipitation situations.
- A review of recent literature, however, reveals that the choice of an appropriate Z-R relationship is not the dominant issue in radar precipitation estimation, as was once widely believed. There are many precipitation estimation errors from radar, and they can often be larger than Z-R irrepresentativeness. These errors are briefly explored as they relate to the WSR-88D. It appears that overshooting of precipitation by the radar beam often produces the largest errors, usually causing precipitation underestimation. This is especially so at long ranges (> 60 nm), but can also occur at short ranges (< 30 nm) because of the beam elevations used by the PPS. Overshoot is more pronounced in the cool season. Inadequate calibration of WSR-88D network radars also appears to contribute large errors.
- Potential remedies for the major errors are reviewed and evaluated for their applicability to the WSR-88D. Recommendations for implementation or further study are made based on this applicability.
1. Introduction
- Radar measurement of surface rainfall has a history almost as long as radar meteorology itself. The radar measures power return, which is expressed as a reflectivity factor Z. This is usually converted to a radar estimate of rain rate, Rr, through an empirical Z-R relationship (Section 4). Surface rainfall is most often measured by rain gages, resulting in a rate Rg. From the weather radar equation, Z is proportional to Di6, where Di are the diameters of individual raindrops in the illuminated sample volume. Rg, on the other hand, is proportional to Di3. This means that the radar measurement is biased toward larger drops. Moreover, different drop size distributions (DSD's) can yield the same Z but different R. This non-unique relationship and the inability (in operational settings) to directly measure the DSD prohibits exact specification of the actual R and precipitation accumulation.
- Even if we knew the DSD precisely, there could be measurement errors by the radar (Z), by the gage (Rg), and by comparison of the two platforms (because of inherent differences in the nature of each measurement). These errors may cause Rr to vary from the true rate R by a typical factor of two (Wilson and Brandes 1979). Correction schemes for Rr may be characterized as analytic (mainly radar-only), statistical/physical, and gage adjustment (Kitchen et al. 1994). This paper reviews the literature concerning the errors, assesses their impact on the WSR-88D Precipitation Processing Subsystem (PPS), and evaluates several correction schemes.
- The PPS was summarized by Hudlow et al. (1991). The PPS was designed to maximize reflectivity data collection pertinent to precipitation calculations. Nevertheless, it introduces errors unique to the PPS algorithm characteristics. This is particularly true of the hybrid scan, which uses Z from one of the lowest four elevation slices. The default hybrid scan, which assumes no obscuring terrain, uses elevations that vary only with range from the radar. They are stepped down with range to collect data near a fixed altitude above site level (ASL), currently 3000 feet. The default scan is subsequently modified for heights of any obscuring terrain and amount of ground return. The hybrid scan is detailed in Shedd et al. (1991).
- Despite its limitations, the PPS is critical to flood forecasts and warnings by the NWS. A recent survey (Lee 1994) showed the one-hour and storm total precipitation (OHP and STP) products to be the most widely used in the entire WSR-88D suite. The literature review and experiences with WSR-88D's in the field suggest opportunities for short and long term improvement of the PPS. Some of these are detailed in this paper.
2. Errors in Radar-Only Estimates
- For thorough discussions of WSR-88D system characteristics, see Heiss et al. (1990), FMH-11 (Part D, 1992), Lemon et al. (1992), and Crum and Alberty (1993). The following lists potential inaccuracies in the measurement of Z, and thus Rr, along with brief explanations. Section 5 elaborates approaches to correct many of these inaccuracies.
a. Radar Calibration
- The WSR-88D calibrates reflectivity every volume scan, using internally generated test signals. From this calibration a "Delta System Calibration" (dB) is calculated. This value reflects change in internal variables such as transmitted power and path loss of the receiver signal processor since the last off-line calibration. If this value becomes large (whereupon an alarm occurs), it is likely that there is a problem with the calibration and precipitation estimates could be significantly in error. It was anticipated that the calibration checks would maintain a reflectivity precision of 1 dB. This translates to an accuracy of 17% in R, using the default Z-R relationship. The present calibration is based on internal RF pulse injection into the receiver. A new calibration procedure was issued in late 1995 (EHB 6 Section 2.7 WSR-88D Maintenance Note 15). It uses instead an external standard RF signal. Several factors may cause Z (and Rr) differences at the same location from adjacent WSR-88D's. One of the more likely factors is a drift in absolute calibration.
- Such Z differences were indeed found for WSR-88D's in Oklahoma by Smith and Krajewski (1994). The differences were much larger than 17%, particularly with heavier rains, and were systematic over long periods. Not only was Rr different, but so was the areal coverage of rain. Ricks et al. (1995) reported average differences of 3 dB between the New Orleans and Mobile WSR-88D's for a rainstorm about equidistant from each site. These authors list several potential causes for this discrepancy. One of them feels that a calibration difference should be added to the list, since it is consistent with the symptoms (J. Graschel, personal communication). Finally, Ulbrich et al. (1996) underscored the need for absolute calibration in a sensitivity study of the Z-R relationship.
b. Attenuation
- The radar corrects for gaseous attenuation, leaving wet radome and intervening precipitation as the principal attenuators of microwave return to and from the target. Both are usually small for S-band. For instance, 60 nm of 50 dBZ rain would only attenuate the received signal 1 dB (Doviak and Zrnic 1984).
c. Frozen Hydrometeors and the Melting Layer
- Rayleigh scattering is assumed, which means that the precipitation particles are presumed small when compared to the wavelength (10 cm for the WSR-88D) of the incident radiation. Further, the weather radar equation is used, which presumes scatterers that are spherical liquid drops, evenly distributed throughout the sampled volume. To describe actual received power that would be received from scatterers meeting the aforementioned constraints, the effective reflectivity factor Ze is introduced in place of Z.
- The most prominent violations of the assumptions come from large frozen hydrometeors - melting snow just below the freezing level (the bright band) and hail. Most studies show a Ze enhancement of 5-10 dB in the bright band, and thus R can be up to five times too large there (Austin 1987; Joss and Waldvogel 1990). Fabry and Zawadzki (1995) found differences up to 16 dB. Thus, the bright band remains a major obstacle to precipitation estimation. The problem is sometimes compounded by the WSR-88D's hybrid scan characteristics, as described in Section 2f, subsection 2.
d. Anomalous Propagation (AP)
- The WSR-88D displays beam heights assuming standard atmospheric refraction, which is rarely the case. Severe deviations from the standard atmosphere occur in layers with large vertical gradients of temperature and/or water vapor. The role of vapor gradients should not be overlooked, since they can substantially change refractivity where there is abundant moisture. This is usually in the lower troposphere and, unfortunately, often accompanies precipitation. Whatever their cause, certain refractivity lapse rates produce superrefraction or subrefraction of the beam and inaccurate calculations of actual beam height. The former is usually the more serious problem, because it can cause ducting or interception of the beam by the ground. This produces persistent and quasistationary returns of high Ze, yielding extreme estimates of (false) precipitation accumulation. This has more operational impact and is more difficult to suppress when AP echoes are imbedded in precipitation echoes. It was anticipated that this would be so severe that, for hydrologic applications at least, further data quality control would be necessary. This is done in Stage II and III precipitation processing, external to the WSR-88D.
- The WSR-88D employs default clutter suppression (notch width and bypass map) and allows operator definition of further clutter suppression regions. The latter capability was designed to diminish temporary clutter such as AP. Several sites, in particular those with complex terrain, have found this feature ineffectual over certain mountains and ridges (e.g., Hitchens et al. 1993). Fig. 1 exhibits AP from the Morristown, TN radar (KMRX), before and after suppression, and its effect on rainfall accumulation. The reduction of higher reflectivities in this instance was only about 5 dB, even with maximum suppression (notch widths). This is typical in the nearby Great Smoky Mountains, where AP imbedded in precipitation echoes has occasionally produced STP amounts exceeding 40 inches (e.g., see Fig. 2). While we have not solved the problem, we have identified the usual AP areas on a Principal User Processor (PUP) background ma to aid recognition as spurious data. This map is overlaid on the upper left quadrant of Fig. 1; the mapped areas correspond well with the actual AP echoes of this event.
- The PPS further attempts to account for AP by the "tilt test." It rejects the lowest tilt (0.5) if areal echo coverage at the tilt just above (1.5) is reduced by an amount greater than that expected from meteorological targets. This value is in adaptation data as "maximum area percent reduction" (MAXPCT) and is currently mandated to be 75%.
e. Beam Blockage
- This is a major problem where radars are situated near mountains, something that is practically unavoidable in many Western U.S. locations. Drastic reductions in the sampled volume by blockage have been illustrated in Switzerland by Joss and Waldvogel (1990). The problem may be mitigated by installing the radar on a peak, but then its lowest elevation slices are so high above valleys (where population is greatest) that near-surface precipitation is not "seen." The PPS software has compensation for up to 60% occultation in the vertical and for 2 or less in azimuth, but many sites have considerable areas with more blockage than this, so no correction is applied. The compensation adds 1-4 dBZe, depending on the percentage of occultation, to reflectivities beyond the blockage for conversion to Rr. The principal uncertainty to this correction is the assumption of standard propagation; under nonstandard conditions the occultation will vary, and so should the amount of correction. This is difficult to quantify in real time.
f. Range Effects
- 1) Elevation of beam - far range
- Earth curvature and standard refraction dictate that the beam becomes more elevated above the surface with increasing range. This effect is akin to blockage, in that the layers near the surface are not sampled by the radar. This is termed beam overshoot or inability to sample the full vertical reflectivity profile. It represents a probability of detection (POD) problem. Fig. 3 shows the reduction in Rr with range for three types of rain. The overshoot will be more pronounced in the cool season, with its lower cloud bases and shallower precipitation. An example of the commonly-observed Rr decline beyond about 90 nm from KMRX is shown in Fig. 2. This figure illustrates not only this problem but also a bright band and AP. All these effects muddled the diagnosis of flood-producing rainfall in southeast Tennessee and western North Carolina.
- Kitchen and Jackson (1993) indicted detection failure as a major cause of underestimated rain accumulation. They found a rapid drop in POD beginning at 60 nm, falling to 0.4 (0.3 in winter) at 124 nm in range (the maximum display range for WSR-88D precipitation products). Interestingly, they suggest that when rain is detected at these far ranges, underestimation of the rate Rr (hence Ze) is smaller than the underestimation of accumulation resulting from low POD. Unfortunately, flooding is related to areal accumulations over a watershed or basin, and radar detection failures will thus lead to underestimates of these.
- 2) Elevation of beam - near range
- Several WSR-88D sites have reported discontinuities in precipitation amounts at constant ranges. The cause for these apparently artificial patterns is uncertain. Smith and Krajewski (1994) documented deficits in WSR-88D precipitation accumulations close to the radar ("holes"), where data originate from higher tilts of the hybrid scan. This may be attributed to software problems or the hybrid scan itself. Fig. 4 shows the default hybrid scan construction for locations without terrain blockage, in which case slices vary only with range. This is not the sectorized scan, whose slices vary also with azimuth to avoid beam blockage by terrain. In the case of low-topped echoes, the holes may be the result of overshoot by the upper hybrid scan slices. This would be exacerbated where radars are situated on mountain tops (mainly in the West) and thus overshoot precipitation in valleys with even the lowest slice.
- The holes could also represent deficits only in a relative sense, when compared to amounts at further ranges. At those ranges, spurious enhancements may be produced by the bright band and/or "bi-scan maximization" (see the next subsection). The latter would be suspect if the hole's maximum range was the same as the minimum range of this maximization, currently about 27 nm. As Ahnert et al. (1983) foretold, the maximization sometimes coincides with the bright band, amplifying the overestimation of Ze and Rr. This artificial enhancement is the reason that the NWS Office of Hydrology (OH) has temporarily suspended the routine. It will be reinstalled in software build 9.0 to allow user specification at ranges beyond the bright band. This build is presently slated for field delivery in Autumn 1996 (T. O'Bannon, personal communication). Even without bi-scan maximization, however, hybrid scan intersections with certain bright band geometries will produce more than one discontinuity with range.
- Smith and Krajewski (1994) ascribe much of the hole phenomenon to software that filters clutter excessively at the higher hybrid scan tilts. This problem is not present at all sites and can be corrected by an adaptation data change (currently in review) and generation of a new clutter filter bypass map under near-standard propagation conditions (Sirmans and Smith 1995). A similar problem will result if a site operator applies suppression for elevation segment 2 (2.5) when there is little clutter or AP at those elevations.
- 3) Beam spreading
- The average WSR-88D half-power beamwidth =0.95. This translates to a beam diameter (r, where r is range to target) of roughly 1 nm at r=62 nm and 2 nm at r=124 nm, the maximum PPS range. The sampled volume quadruples for every doubling of range. This resolution degradation also limits detection of severe weather signatures (e.g., Hunter et al. 1993). It increases the likelihood that precipitation fills only part of the beam. Since it is assumed that scatterers fill the sample volume completely and uniformly, one may expect sample volume averaging of received power to yield reduced reflectivity over that of a nearer volume. It will weaken the bright band but distort it in the vertical (Fabry et al. 1992). Spreading will also cause overestimation of echo top height.
- A range degradation correction to Rr is included in the adaptable parameters of the Radar Product Generator (RPG); this is currently disabled, pending scientific data to support accurate parameterization. There is another algorithm that is presently implemented, however, which impacts Rr at longer ranges. It is the bi-scan maximization. It selects the maximum Ze of the lower two tilts 1 and 2 (Fig. 4) for precipitation processing at each bin, unless the tilt 1 value has been thrown out by the tilt test. In the latter case, tilt 2 is always used. Maximization was originally invoked to account for pitch and roll of a shipborne research radar, but was also expected to compensate for beam blocking and nonstandard refraction in the WSR-88D (Ahnert et al. 1983). It has the added desirable effect of counteracting Ze losses with range (Shedd et al. 1989).
3. Radar vs Rain Gage Estimates
a. Rain Gages
- Because of its long service and widespread use, the rain gage has become the standard for measuring surface rainfall and is often assumed to be "ground truth" in studies using other measurement technologies, such as radar. The validity of this assumption is undermined by several errors in gage measurement. Since these are well known, only brief mention will be made here. They include wind/turbulence losses and tipping bucket losses with high rainfall rates (Alena et al. 1990). The wind/turbulence errors are usually around 5% but can be as large as 40% in high winds, such as with thunderstorm outflows (Wilson and Brandes 1979).
- Although gage accuracy is usually high, the main problem is that the measurement is for essentially a point, compared to a radar sample volume that yields rainfall over a much larger area. The latter is more relevant to hydrology, in which rain over a fixed catchment area is desired. To facilitate comparison between radar (Rr) and gage (Rg), many experiments have used networks of gages. This too has limitations, as subsequently related.
b. Radar/Gage Sampling Differences
- The customary use of gage data is to "adjust" Rr through various statistical techniques, so that the (usually) superior accuracy of Rg is applied to the greater areal coverage of radar Rr. The integration of these two types of data is problematic. The temporal sampling of the WSR-88D is every 5 or 6 minutes; for gages it is nearly continuous (although data communication to an office often lags). More important is the spatial disparity of the two measurements. Not only is the radar sample volume larger, as stated before, but it is elevated above the gage. The more elevated the beam, the more likely Rr will deviate from Rg, which is of course from the desired ground level. Thus, the overshoot problem cited in Section 2f is an extreme example of this discrepancy. Physical processes such as evaporation, coalescence, and precipitation displacement by horizontal wind may become important with increasing overshoot.
- Even if the beam is sufficiently low to supply a good estimate of the surface precipitation rate over a gage, how does one apply the Rg/Rr ratio at this point (and others) to the remainder of the Rr field? This question has been the subject of many studies. Brandes (1975) proposed using data from several gage locations to obtain a single calibration factor (average Rg/Rr) or bias that is applied to the entire Rr field. This should make the total field volumetric water estimate match the true value. Ahnert et al. (1983) used a similar scheme but passed the ratios through a Kalman filter. This is incorporated into the PPS rain gage (bias) adjustment algorithm. This part of the algorithm should be implemented with the supporting Gage Data Support System (GDSS).
- The effectiveness of this technique is in direct proportion to the sampling effectiveness of the gage network. There must be a sufficient number of gages to faithfully represent the rainfall field under the radar umbrella. POD is also increased by uniformity in gage spacing (Grosh 1993).
- Finally, the technique implicitly assumes that the bias is uniform under the umbrella. If these conditions are not met, gage adjustment may degrade the accuracy of the Rr field in localized areas (Lin and Krajewski 1990; Zawadzki 1984). This phenomenon is more probable during isolated, convective precipitation, where gages are less likely to be under precipitation shafts, or they may lie under large reflectivity gradients. Also, the bias will be spatially variable when the near-range and far-range effects of Sec. 2f impair Rr values. Unfortunately, it is hard to distinguish sampling from physical contributions to Rg/Rr differences. (Koistinen and Puhakka 1984).
4. Z-R Relationships
- These relationships vary from one experiment to the next, and thus number in the hundreds (Battan 1973 lists 69). The variation is caused by the ambiguity in drop size distribution (DSD), as broached in Section 1. The DSD is determined by a complex interaction of microphysical processes. It fluctuates daily, seasonally, regionally, and even within the same cloud. Add to this variability the aforementioned uncertainties of radar estimates of Rr, and the magnitude of the problem becomes great. All Z-R relations assume the same things as in the calculation of Ze, notably that the scatterers are liquid drops that are small compared to the radar wavelength. These are particularly troublesome assumptions, as bright band and hail contamination are common.
- The WSR-88D's Z-R is part of adaptation data, and is expected to be tailored according to the above influences. The default is Z=300 R1.4, which is a compromise between stratiform and convective relationships. This relation produces a doubling/halving of R with a difference of only ±4 dBZe! Given such sensitivity, how does one adequately quantify each influence?
- The good news concerning this task is that the choice of Z-R relation does not affect the outcome as much as the Z measurement itself. Fig. 5 depicts some common Z-R relations. All but one of the curves (the warm orographic rain of Blanchard) are close to the well-known Marshall-Palmer relation, and this is true of most curves catalogued by Battan (1973). For the three close curves in this figure and 40 dBZ (Z=104 mm6 m-3), their range in R is only 0.3"/hr. Interestingly, Branick and O'Bannon (1993) appealed to the outlying Blanchard relation to explain WSR-88D underestimation (by about 50%) of storm total rainfall behind a cold front. Such an appeal appears to have little physical foundation, and this will be discussed further in Section 5b, subsection 1.
- Further illustration of Z vs Z-R sensitivity is offered in Fig. 6. Rain was captured over three seasons in a disdrometer, which measures DSD. In both 6(a) and 6(b), R was calculated from that DSD and plotted logarithmically on the ordinate. In (b), the abcissa represents Z calculated from the same disdrometer data; in (a), Z is estimated from a nearby radar. Scatter about a fixed Z-R line in the disdrometer-only plot (b) is from Z-R relationship variations only, while scatter in the radar-measured Z plot (a) is from those plus radar sampling differences. Scatter in (a) is triple that in (b)(Zawadzki 1984). This increased uncertainty in R resulted despite relatively ideal radar geometry (r = 19 nm, beam elevation = 360 m). Therefore radar/gage sampling differences, discussed in Section 3a, can overwhelm differences owing to an irrepresentative Z-R (Fabry et al. 1992). Zawadzki (1984) and Smith (1990) agree, saying that DSD (thus Z-R) variations do not present the chief obstacle to radar precipitation measurement.
- Furthermore, Z-R selection errors are mitigated by gage bias adjustment and averaging of Rr over large time and space scales (Hudlow and Arkell 1978; Ahnert et al. 1983). Such beneficial averaging may be realized by methods like the area-time integral (ATI) of Doneaud et al. (1984). The ATI tracks rainfall duration and area (exceeding a prescribed Ze threshold) rather than a detailed Ze field.
5. Improvements to Radar Precipitation Estimates
a. Near-term solutions
- There are several approaches that might offer some short-term remedies to Rr errors. Several employ functionality that already exists in the WSR-88D system.
- 1) Tilt test modification
- The tilt test, detailed in Section 2d, was devised to eliminate AP contamination of precipitation estimates from the lowest tilt. Unfortunately, the test can reject the lowest tilt when it contains valid meteorological echoes. This is more common with shallow stratiform precipitation, in which there is often a pronounced decrease in echo coverage from the lowest tilt to the one just above. The lowest tilt is especially crucial to precipitation estimation at the distant ranges where the tilt test is now applied (nominally > 30 nm), since all tilts are at relatively high altitudes there (Fig. 4). They are even higher at mountain-top radar sites (Sec. 2f).
- An obvious improvement is to ensure that the tilt test does not reject the lowest tilt in non-AP situations. This may be accomplished by increasing the maximum area percent reduction (MXPCT) in adaptation data. The NWS OH has recently directed such an increase, from 50% to the maximum 75% (R. Fulton, personal communication). If a site continues to experience lowest tilt rejection with this new threshold, we should consider the more drastic option of disabling the tilt test entirely. This is possible at present via alteration of other adaptation data, and via a UCP command in the next software build, 9.0.
- The higher percentage, on the other hand, may allow residual AP to pass as precipitation more often. Forecasters should be on guard for these cases and reduce MAXPCT (in adaptation data) accordingly, after they've attempted to suppress the AP via clutter suppression regions.
- 2) Bi-scan maximization
- Bi-scan maximization compensates for range degradation in the right direction, i.e., Ze adjustments are always positive. But the algorithm was not originally designed to alleviate range degradation and, since it simply chooses a maximum Ze from the two lowest tilts, it has an uncertain quantitative physical or statistical foundation. Moreover, it occasionally causes overestimation of Rr, especially when coincident with the bright band. Thus it is being reimplemented in Build 9.0 at ranges unlikely to be affected by the bright band (Seo et al. 1995).
- The "true" range degradation correction in adaptation data appears far from activation. Yet if range degradation of Ze and Rr is indeed less significant than the POD problem (Kitchen and Jackson 1993), the need for additional correction would be diminished.
- Since the bi-scan maximization can act as a surrogate correction, perhaps it is sufficient for this purpose. To ascertain if this is the case, its effects should be studied locally in cases of heavy precipitation. This can be done by examination of archived WSR-88D data, with software that can enable and disable the bi-scan maximization. An example of such software is the Radar Analysis and Display System (RADS, Sanger 1994; now called WATADS). This has been provided for use on the Science Applications Computer at Weather Forecast Offices (WFO's).
- 3) Radar calibration
- The reports of systematic Ze differences between nearby WSR-88D sites, as cited in Section 2a, indicate that radar calibration is a serious problem and needs immediate attention. First, the 1995 calibration mentioned in that section should be done periodically and systematically by all sites. This requires that all sites have the proper test equipment. Then Ze comparisons between adjacent radars should be made to ensure that differences do not exceed 1 dB. If they remain outside this tolerance, the radar calibration(s) can be "tuned" to be within it. This will be tested for KMRX and adjacent radars. A network-wide plan for such calibration is desirable.
- 4) Hail threshold
- The PPS does not process Ze above a certain adaptable threshold, based on the supposition that such reflectivities are due to hail and not rain. The present threshold is set to 53 dBZe, but sites may seek to change it based upon climatological regime. For example, in the cooler regime of Colorado, WSFO Denver uses 50 dBZe. For the warm climate of Florida, NWSO Melbourne plans to use 55 dBZe. Sites that experience consistently low (high) Rr with convective storms should gather hail and climatological data to support an increase (decrease) in the threshold.
- 5) Averaging methods
- Errors in Rr owing to an irrepresentative Z-R relationship should diminish when greater temporal and spatial averaging is done, as discussed in Section 4. Averaging is also more applicable to hydrologic runoff calculation, which typically employs (gage-derived) mean areal precipitation (MAP) over a fixed catchment. River Forecast Centers (RFC's) already calculate MAP from incoming PPS data; it is recommended that the WSR-88D include an algorithm to generate MAP products for the WFO. The ATI methods mentioned earlier could serve as a starting point for such algorithm development, as might a technique described by Davis (1993). He has shown that the Average and Maximum Basin Estimated Rainfall (AMBER) flash flood warning system can provide critical lead time for small (~30 km2) basins, once they are defined. This capability would take full advantage of the high resolution of WSR-88D reflectivity and precipitation data.
- 6) Modified hybrid scan/elevation angles
- Radar precipitation measurement should sample as close to the surface as possible, yet avoid deterioration from ground clutter, AP, or blockage. This was the intent of the hybrid scan. There are opportunities for improvement on the present scan. First, it may be constructed with a different combination of existing elevation slices. If the near-field slices (3.4 and 2.4) consistently overshoot shallow precipitation and result in "holes" (Sec. 2f), then lower slices may offer improvement. Second, if the deteriorating phenomena listed above can be minimized, additional low slices should be incorporated in the WSR-88D's volume coverage patterns (VCP's). This involves more study than the first option, since VCP modification will affect other algorithms, VCP duration and dwell times, etc. If adverse effects result, they would have to be weighed against improvements in precipitation estimation. If there is a net benefit and the change is undertaken, it may use an existing proposal. This is shown in Fig. 7 (compare to Fig. 4). It lowers the average constant height of measurement from 3,000 to 2,500 feet ASL by adding an elevation at 1.0. Further reduction of the constant height has been recently proposed by OH, via their optimized hybrid scan (Breidenbach et al. 1995). This scan constructs a constant altitude PPI (CAPPI) based on a climatological vertical reflectivity profile and the VCP.
- 7) Gage Data Support System (GDSS)
- The WFO will, with assistance from its servicing RFC, select up to 50 rain gages for polling by the RGDAF and subsequent bias calculations. Gage locations should be as close as possible to the ideals of uniform spacing and comprehensive coverage of the radar umbrella, per the description in Section 3a. Since the bias calculation will be impaired by inclusion of Rg/Rr pairs where Rr is degraded by near and far-range effects, Seo et al. (1995) recommended a range-dependent exclusion of pairs from the calculation. This should accommodate exclusion of pairs from a bright band region as well.
- Forecasters should understand limitations of the bias calculation in various weather situations, so they can determine the effect of the bias adjustment on the overall Rr field. Until pair exclusion is implemented, the entire adjustment should be temporarily disabled in cases of Rr degradation. This capability already exists at the Unit Control Position (UCP). Longer-term solutions in the form of alternatives or modifications to the Kalman filter scheme are explored in the following section.
b. Long-term solutions
- These solutions are classified as long-term because they are currently external to the WSR-88D, and generally drawn from the research community. They would require substantial study on their merit and feasibility as WSR-88D algorithms before incorporation.
- 1) Z-R relationship
- This relationship is easily changed in adaptation data, per the expectation of system developers. It is difficult to find sound scientific rationale for changing Z-R, however, especially when faced with operational time and data constraints. Fluctuations of DSD are often so rapid and localized that it is nearly impossible to select a single representative Z-R relation for the entire radar umbrella, or for any length of time.
- There are some instances, for example long-lived and widespread stratiform rain events, where the DSD is stable enough to be characterized by a single Z-R relation. These instances must be identified by considerable local research, defining the relationship for each precipitation system in question. This has been done for one system type by the WSR-88D Operational Support Facility (OSF) - see subsection 4 below. Even in these cases, however, forecasters should be aware that DSD variation, which a Z-R relation attempts to characterize, may not be the principal influence on precipitation estimation uncertainties (Sec. 4). Given a knowledge of all the major influences on Rr presented in this paper, he or she should be prepared to at least use qualitative reasoning to reinterpret the precipitation estimates furnished by the WSR-88D.
- 2) Bright band corrections
- Correction algorithms have been advanced by Cheng and Collier (1993), Smith (1986), and Kitchen et al. (1994). The latter two identify the bright band by its pronounced vertical discontinuity in Ze. This vertical profile is then modeled, from which a "background" Ze is calculated. This value is proposed as that which would exist in the absence of the bright band enhancement. Both methods are the subject of continuing research by NWS OH for possible inclusion into the WSR-88D (Seo et al. 1995). This research indicates that much additional data analysis is required before any such scheme is implemented.
- This conclusion is supported by the findings of Fabry and Zawadzki (1995), who report larger variability in bright band intensities than previously known. They add that even the physical cause of bright band enhancement is still open to debate, as the classical explanation of large water-coated snow aggregates could only account for 50% of their observations. Such uncertainties complicate the development of robust correction techniques.
- 3) AP corrections
- The seriousness of AP contamination has given impetus to a high-priority NWS investigation of AP rejection algorithms (Interagency MOU 1995). Weber et al. (1993) and Moskowicz et al. (1994) recently presented identification/rejection methods that could provide a foundation for development of WSR-88D algorithms. Such methods are needed to combat loss of credibility by users of PPS products.
- 4) Gage data adjustment techniques
- This field embraces a large array of procedures, several originating as far back as the 1970's. Most are statistical, but there are wide variations in analysis mode. The sampling problems addressed in Section 3a affect all of them. Whichever one is used in the bias adjustment algorithm should minimize these problems as much as possible for estimation of heavier rainfall, which is of primary concern in flood forecasting.
- The bias adjustment algorithm will eventually be activated in the WSR-88D. It has recently been tested in a few cases and was shown not to degrade the Rr field, even with input from as little as three gages (Seo et al. 1995). It will continue to be evaluated in the field, however, and might be changed or simplified if problems are found. If its benefits do not outweigh its liabilities, the scheme should be overhauled, augmented, or replaced. A few prototypes for potential augmentation or replacement follow.
- Modification of adjustment for specific meteorological regimes or physical factors has been done by Collier et al. (1983) and Austin (1987). Classification of a precipitation system as stratiform or convective should be feasible at the very least.
- A good candidate for classification might be "tropical" systems. There is much evidence that the PPS underestimates rainfall with these systems (Natural Disaster Survey Report 1995; Ruthi et al. 1993; Hitchens et al. 1993, Woods et al. 1995). This may result from their high precipitation efficiencies, DSD's weighted toward small sizes or, less likely, from a hail threshold that is too small for these cases. In response to the evidence, the OSF recently submitted a new relation, for use in the field during "tropical rain" events. This relation, Z=250 R1.2 (from Rosenfeld et al. 1993), provided good Rr estimates in four tropical rainstorms in Texas and Florida. It will be interesting to follow the performance of this relation upon a larger sample of tropical systems, across the country.
- Finally, Rosenfeld et al. (1994) proposed that Rr be adjusted locally, in small windows surrounding a gage. This requires more computation than for a single bias, but not enough to tax the workstations of today. A bigger obstacle is a sparsity of gages under many WSR-88D umbrellas.
- 5) Vertical reflectivity profile (VRP) corrections
- This category could also be termed "range dependent corrections," since the portion of the VRP observed by a radar is determined by its range. The bright band strongly affects the shape of the VRP. The layer it occupies is normally well enveloped within radar coverage however, so corrections to the bright band itself are treated in a separate subsection (2). The bright band height divides layers with very different Ze profiles. The sampling of these profiles is range dependent and so is discussed later in this subsection. An even more serious consideration are those effects that prevent the radar from "seeing" the lower portion of the VRP, the most representative portion for precipitation estimation. These are beam blockage (Sec. 2e) and elevation (Sec. 2f).
- Joss & Waldvogel (1990) assert that VRP measurement is "...the main problem in using radar for precipitation measurements and hydrology in operational applications." This is affirmed by several researchers, including Koistinen (1991), Galli and Joss (1991), Andrieu and Creutin (1991), and Smith (1990). Joss and Waldvogel (1990) reinforce the importance of VRP correction by asserting that it should be done before any other adjustment, as with gage data.
- The VRP problem has not received proper attention because it does not usually arise in research experiments. These experiments generally measure DSD and Ze at medium ranges (20 nm < r < 60 nm) of the radar, over areas without terrain obstruction. Thus, range effects are minimized. Such a luxury is not possible with an operational radar such as the WSR-88D. As noted earlier, far-range effects begin to impair Rr at about 60 nm, yet the PPS produces estimates to 124 nm. PPS products from adjacent radars usually do not have overlapping coverage, since the average spacing of the WSR-88D network is 136 nm.
- VRP obscuration generally leads to underestimation of Rr, whether its cause is blockage, near-range or far-range effects. Both range effects are at work in the PPS, as documented in Smith et al. (1996). They found underestimation (relative to gages) at all ranges, but it was most pronounced at r < 30 nm and r > 60 nm. This produces an apparent maximum at the intermediate ranges (30 nm < r < 60 nm), which may be compounded by overestimation from the bright band.
- Corrections to the problem are complicated, because questionable assumptions are necessary to correct for what cannot be seen. Some correction methods are discussed in the remainder of this section. The range degradation correction in the WSR-88D was designed to compensate for beam spreading, not beam overshoot, and is therefore not applicable to this problem.
- For beam blocking and overshoot, there have been various methods for synthesizing a VRP to substitute for the unseen part of the profile. Gray (1991) experimented with an Eigenvector formulation. Koistinen (1991) obtained daily average profiles from echoes relatively close to the radar. Joss and Pittini (1991) also calculated daily profiles, but adjusted them with gage data. Joss and Waldvogel (1989) used seasonally averaged profiles. They, along with Koistinen (1986), maintain that even a crude estimate of VRP can significantly improve Rr. Nevertheless, they recommend application of a different correction for every pixel, based on the elevation of the lowest radar sample above the underlying terrain. This specifies the vertical extent of the synthesized VRP at each pixel location. The WSR-88D's occultation file is generated from USGS terrain data, so this part of the VRP correction should not be difficult to synthesize.
- Finally, there are VRP models based on physical reasoning. The VRP is usually represented as relatively constant in the rain below the bright band and sharply decreasing in snow above (e.g., Smith 1986; Kitchen et al. 1994). Sampling of only the snow layer leads to serious underestimation of Rr and represents a transition toward complete beam overshoot. Indeed, increasing range/beam elevation acts with a low bright band to reduce Rr to the point of uselessness. Fabry et al. (1992) used this fact to calculate a maximum usable range for Rr that is proportional to bright band height. Even for a relatively high cool-season bright band of 3 km (10 kft), they calculated a maximum range of only 65 nm in stratiform rain.
- This disappointing result is countered by others who have synthesized a snow VRP in an attempt to obtain a correction. This was done using climatological VRP data by Kitchen et al. (1994), yielding increased accuracy in heavy rain cases particularly. From his daily mean VRP's with a bright band present, Koistinen (1991) derived a linear range-dependent correction (dB) based on the height of the freezing level. Similar local data sets could be obtained from a WSR-88D, offering hope for a correction. The bright band and adjoining VRP varies considerably (Fabry and Zawadzki 1995) or the bright band may not exist at all, so care must be taken in local data collection and application.
- Finally, physical processes can significantly alter the lower (rain) VRP. One such process is low-level precipitation enhancement by orography, which was addressed by Hill (1983).
6. Summary and Recommendations
- The last section touched on a few alternatives or enhancements to the PPS. When evaluating these and other techniques, one should keep in mind the strengths and weaknesses of the present PPS and the principal applications of precipitation products within the NWS (including RFC's). For WFO's, the chief application is flood forecasting and so light rain is of less concern than heavy rain. The present PPS scheme takes advantage of the high temporal and spatial resolution of the WSR-88D to locate "bullseyes" of heavy rain very well. So the PPS of today is of great value and represents a tremendous leap forward in flood forecasting compared to previous radars and gage-only networks. The potential for improvement is with the magnitudes of those bullseyes, since these can be in error by a factor of two or more.
- To this end, the most promising methods should have at least two desirable traits. First, they should be straightforward and relatively easy to implement in an operational system. This tilts the deliberation toward the near-term solutions of section 5a. Second, they should take into account not only radar characteristics but the meteorological knowledge of NWS forecasters. This knowledge is indispensable and should embrace physical impacts on precipitation such as vertical motion (orographic or otherwise), horizontal wind, drop breakup and evaporation, coalescence, hail, etc. (e.g., Austin 1987; Zawadzki 1984). The classification of tropical systems in Sec. 5b (subsection 4) exemplifies a first step in such physical thinking, which will hopefully transcend simply searching for a Z-R relationship that "fits." Statistical methods have predominated in the literature but physical considerations, even when difficult to quantify, should prove an increasingly valuable modulator of Rr estimates in the future.
- Although a few radars will be added to the WSR-88D network per the National Research Council's (1995) recommendations, these will not augment coverage enough mitigate the range effects described in section 2f. These impacts are the most serious ones at far ranges from the radar (> 60 nm). The best way to mitigate these effects is to maximize valid data collection from the lowest tilts. Given that AP elimination is adequate (a major assumption), this can done by modified hybrid scans. Proposals for such scans already exist, as represented by Fig. 7 or by the CAPPI technique of Breidenbach et al. (1995). Modification of existing VCP's may be a viable option. A suite of hybrid scans, adaptable to various precipitation events, could pose a longer-term solution.
- In very far-range regions of overshoot by even the lowest tilt, VRP insertion appears to offer the most hope for Rr improvement. Even a climatological VRP can increase accuracy substantially. Archive level II data from the WSR-88D can be a source for generation of such climatological profiles.
- Adjustment of the Rr field by gage data, via the GDSS and Kalman filter, is still of questionable value in some cases. Preliminary tests of the algorithm suggest that it improves estimates with input from just a few gages. Nevertheless, there are wide variations in gage density and placement across the country, so much more study is needed. WFO's should thus conduct local studies on the effect of this adjustment under various meteorological conditions. These studies will help researchers tune, augment, or replace the adjustment as warranted.
- Calculation of Mean Areal Precipitation (MAP) over predefined basins by the WSR-88D should be given immediate high priority. This calculation should be relatively simple to implement and is the most relevant to hydrologic models and flood forecasts. The AMBER system described by Davis (1993) is already in use at WFO Pittsburgh and thus would be an attractive model for such calculation. Efforts are underway (R. Davis, personal communication) to allow AMBER to use a new Build 9.0 product, Digital Hybrid Scan Reflectity (DHR). A simpler, interim step would be to provide background maps of sub-basins at the PUP.
- Local hail reflectivity thresholds should also be investigated, and most of this can be done at the local WFO. Local research into Z-R relationship modification should be continued but deemphasized. As to long-term solutions, precedence should be given to research into AP and bright band suppression.
- As indicated by the survey of field personnel (Sec. 1), there is already great utility in the current suite of PPS products at WFO's. The recommendations made here may enhance their utility and the author urges that they be given serious consideration by NEXRAD program organizations, particularly the NWS Office of Systems Operations, Office of Meteorology, WSR-88D OSF, and NWS OH. The same is hoped for agencies developing the new Advanced Weather Interactive Processing System (AWIPS), since WSR-88D products will soon be processed and viewed at AWIPS workstations at WFO's.
ACKNOWLEDGMENTS
- This paper was begun while I attended the COMET Mesoscale Analysis and Prediction class in Boulder, CO. I am grateful to COMET for providing the facilities and support for launching the project. I am particularly indebted to Roger Pierce of OH/COMET, with whom I worked closely during the germinal stage. Dr. James Smith (Princeton Univ.), Rich Fulton (OH), Tim O'Bannon (WSR-88D Operational Support Facility), Paul Jendrowski (WSFO PIT), Jeff Graschel (Lower Mississippi RFC), and Matt Kelsch (NOAA/Forecast Systems Lab) supplied much valuable information.
REFERENCES
- Ahnert, P.R., M.D. Hudlow, E.R. Johnson, and D.R. Greene, 1983: Proposed "on-site" precipitation processing system for NEXRAD. Preprints, 21st Conf. Radar Meteor., Amer. Meteor. Soc., Boston, 378-385.
- Alena, T.R., J.S. Appleton, and W.H. Serstad, 1990: Measurement accuracy of tipping bucket rain gauges at high rainfall rates. Preprints, Conf. on Operational Precipitation Estimation and Prediction, Amer. Meteor. Soc., Boston, 16-19.
- Andrieu, H., and J.D. Creutin, 1991: Effect of the vertical profile of reflectivity on the rain rate assessment at ground level. Preprints, 25th Intl. Conf. Radar Meteor., Amer. Meteor. Soc., Boston, 832-835.
- Atlas, D., and C.W. Ulbrich, 1974: The physical basis for attenuation-rainfall relationships and the measurement of rainfall parameters by combined attenuation and radar methods. J. Rech. Atmos., 8, 275-298.
- Austin, P.M., 1987: Relation between measured radar reflectivity and surface rainfall. Mon. Wea. Rev., 115, 1053-1071.
- Battan, L.J., 1973: Radar Observation of the Atmosphere. The Univ. of Chicago Press, 324 pp.
- Brandes, E.A., 1975: Optimizing rainfall estimates with the aid of radar. J. Appl. Meteor., 14, 1339-1345.
- Branick, M., and T. O'Bannon, 1993: Analysis of WSR-88D rainfall estimates during a widespread heavy rainfall event in Oklahoma. NWS Southern Region Technical Attachment SR/SSD 93-44, 8 pp. Ft. Worth, TX.
- Breidenbach, J.P., D.J. Seo, R. Fulton, and D. Miller, 1995: Improving WSR-88D precipitation estimates through optimization of the hybrid scan. Preprints, 27th Intl. Conf. Radar Meteor., Amer. Meteor. Soc., Boston, 243-245.
- Cheng, M., and C.G. Collier, 1993: An objective method for recognizing and partially correcting brightband error in radar images. J. Appl. Meteor., 32, Amer. Meteor. Soc., Boston, 1142-1149.
- Collier, C.G., P.R. Larke, and B.R. May, 1983: A weather radar correction procedure for real-time estimation of surface rainfall. Quart. J. Roy. Meteor. Soc., 109, 589-608.
- Crum, T.D., and R.L. Alberty, 1993: The WSR-88D and the WSR-88D Operational Support Facility. Bull. Amer. Meteor. Soc., 74, 1669-1687.
- Davis, R.S., 1993: AMBER A prototype flash flood warning system. Preprints, 13th Conf. Weather Analysis and Forecasting, Amer. Meteor. Soc., Boston, 379-383.
- Doneaud, A.A., S. Ionescu-Niscov, D.L. Priegnitz, and P.L. Smith, 1984: The area-time integral as an indicator for convective rain volumes. J. Climate Appl. Meteor., 23, 555-561.
- Doviak, R.J., and D.S. Zrnic, 1984: Doppler Radar and Weather Observations, Academic Press, San Diego, 458pp.
- Fabry, F., G.L. Austin, and D. Tees, 1992: The accuracy of rainfall estimates by radar as a function of range. Quart. J. Roy. Meteor. Soc., 118, 435-453.
- ____, and I. Zawadzki, 1995: Long-term radar observations of the melting layer of precipitation and their interpretation. J. Atmos. Sci., 52, 838-851.
- Federal Meteorological Handbook (FMH) No. 11, 1992: Doppler Radar Meteorological Observations, Part D. Office of the Federal Coordinator for Meteorological Services and Supporting Research FCM-H11D-1992, Washington, D.C.
- Galli, G., and J. Joss, 1991: Using and adjusting conventional radar reflectivity data for estimation of precipitation: past, present and future studies in Switzerland. Hydrological Applications of Weather Radar, I.D. Cluckie and C.G. Collier Eds. Ellis Horwood, Chichester England, 65-73.
- Gray, W.R., 1991: Vertical profile corrections based on EOF analysis of operational data. Preprints, 25th Intl. Conf. Radar Meteor., Amer. Meteor. Soc., Boston, 821-823.
- Grosh, R.C., 1993: Radar rain measurement calibration networks. Preprints, 13th Conf. Wea. Anal. and Forecasting, Amer. Meteor. Soc., Boston, 401-402.
- Heiss, W.H., D.L. McGrew, and D. Sirmans, 1990: NEXRAD: Next generation weather radar (WSR-88D). Microwave J., Jan., 79-98.
- Hill, F.F., 1983: The use of average annual rainfall to derive estimates of orographic enhancement of frontal rain over England and Wales for different wind directions. J. Climatology, 3, 113-129.
- Hitchens, R.D., J.L. Wiesmuller, and S.M. Zubrick, 1993: WSR-88D precipitation estimates an overview. Preprints, 13th Conf. Wea. Anal. and Forecasting, 367-372.
- Hudlow, M., and R. Arkell, 1978: Effect of temporal and spatial sampling errors and Z-R variability on accuracy of GATE radar rainfall estimates. Preprints, 18th Conf. Radar Meteor., Amer. Meteor. Soc., Boston, 342-349.
- Hudlow, M., J.A. Smith, M.L. Walton, and R.C. Shedd, 1991: NEXRAD: new era in hydrometeorology in the USA. Hydrological Applications of Weather Radar, I.D. Cluckie and C.G. Collier Eds. Ellis Horwood, Chichester England, 602-612.
- Hunter, S.M., 1993: A limiting case for the WSR-88D - a severe "gustnado." Preprints, 26th Conf. Radar Meteor., Amer. Meteor. Soc., Boston, 660-663.
- Interagency Memorandum of Understanding (MOU) among the NEXRAD program, Operational Support Facility, and the National Weather Service Office of Hydrology, 1995.
- Joss, J., and A. Waldvogel, 1989: Precipitation estimates and vertical reflectivity profile corrections. Preprints, 24th Conf. Radar Meteor., Amer. Meteor. Soc., Boston, 682-688.
- ____, and ____,1990: Precipitation measurements and hydrology. Radar in Meteorology, D. Atlas Ed. Amer. Meteor. Soc., Boston, 577-606.
- ____, and A. Pittini, 1991: The climatology of vertical profiles of radar reflectivity to improve estimates of precipitation. Preprints, 25th Intl. Conf. Radar Meteor., Amer. Meteor. Soc., Boston, 828-831.
- Kitchen, M., R. Brown, and A.G. Davies, 1994: Real-time correction of weather radar data for the effects of bright band, range and orographic growth in widespread precipitation. Quart. J. Roy. Meteor. Soc., 120, 1231-1254.
- ____, and P.M. Jackson, 1993: Weather radar performance at long range - simulated and observed. J. Appl. Meteor., 32, 975-985.
- Klazura, G.E., 1995: Adaptable parameter changes to improve WSR-88D tropical rainfall estimates. WSR-88D Operational Support Facility memo, 10/6/95.
- Koistinen, J., 1986: The efffect of some measurement errors on radar-derived Z-R relationships. Preprints, 23rd Conf. Radar Meteor. and Cloud Physics Conf., Amer. Meteor. Soc., Boston, JP50-JP53.
- ____, 1991: Operational correction of radar rainfall errors due to the vertical reflectivity profile. Preprints, 25th Intl. Conf. Radar Meteor., Amer. Meteor. Soc., Boston, 91-94.
- ____, and T. Puhakka, 1984: Can we calibrate radar by raingauges. Preprints, 22nd Conf. Radar Meteor. and Cloud Physics Conf., Amer. Meteor. Soc., Boston, 263-267.
- Lee, R.R., 1994: Survey results of WSR-88D field sites meteorological algorithm performance, 1994. Postprints, First WSR-88D Users' Conference, WSR-88D Operational Support Facility, Norman, OK, 1-8.
- Lemon, L.R., E.M. Quoetone, and L.J. Ruthi, 1992: WSR-88D: Effective operational applications of a high data rate. Preprints, Symposium on Weather Forecasting, Amer. Meteor. Soc., Boston, 173-180.
- Lin, D., and W.F. Krajewski, 1990: A recursive algorithm to adjust biased radar rainfall fields. Preprints, Conf. on Operational Precipitation Estimation and Prediction, Amer. Meteor. Soc., Boston, 103-107.
- Moskowicz, S., G.J. Ciach, and W.F. Krajewski, 1994: Statistical detection of anomalous propagation in radar reflectivity patterns. J. Atmos. Oceanic Tech., 11, 1026-1034.
- National Research Council (NRC), 1995: Toward a New National Weather Service - Assessment of NEXRAD Coverage and Associated Weather Services. National Academy Press, Washington D.C., 104 pp.
- Natural Disaster Survey Report, 1995: Southeast Texas Tropical Mid-Latitude Rainfall and Flood Event, October 1994. NWS Southern Region, Ft. Worth, TX.
- Richards, W., and C. Crozier, 1983: Precipitation measurements with a C-band radar in southern Ontario. Atmos.-Ocean, 21, 125-137.
- Ricks, R., J. Graschel, and E. Jones, 1995: A comparison of adjacent WSR-88D precipitation estimates along the Mississippi Gulf Coast. NWS Southern Region Technical Attachment SR/SSD 95-26, 6 pp. Ft. Worth, TX.
- Rosenfeld, D., D.B. Wolff, and D. Atlas, 1993: General probability-matched relations between radar reflectivity and rain rate. J. Appl. Meteor., 32, 50-72.
- ____, ____, and E. Amitai, 1994: The window probability matching method for rainfall measurements with radar. J. Appl. Meteor., 33, 682-693.
- Ruthi, L.J., E.M. Quoetone, and L.R. Lemon, 1993: Operational analysis of selected meteorological phenomena with the WSR-88D. Preprints, 13th Conf. Wea. Anal. and Forecasting, Amer. Meteor. Soc., Boston, 469-476.
- Sanger, S., 1994: An interactive Doppler radar and weather detection algorithm display system. Preprints, 10th Intl. Conf. on Interactive Information and Processing Systems for Meteorology, Oceanography, and Hydrology, Amer. Meteor. Soc., Boston, 7-11.
- Seo, D.J., R. Fulton, J. Breidenbach, D. Miller, and E. Friend, 1995: Final Report for October 1 1993 to October 31 1994, Interagency Memorandum of Understanding among the NEXRAD program, Operational Support Facility, and the National Weather Service Office of Hydrology Hydrologic Research Laboratory. Hydrologic Research Laboratory, NWS Office of Hydrology, Silver Spring MD.
- Shedd, R.C., J.A. Smith, and M.L. Walton, 1989: Sectorized hybrid scan strategy of the NEXRAD precipitation processing system. Preprints, Intl. Symp. Hydro. Applications of Wea. Radar, Univ. of Salford, Salford, England, 9 pp.
- ____,____, and ____, 1991: Sectorized hybrid scan strategy of the NEXRAD precipitation-processing system. Hydrological Applications of Weather Radar, I.D. Cluckie and C.G. Collier Eds. Ellis Horwood, Chichester England, 151-159.
- Sirmans, D., and S. Smith, 1995: Investigation of reflectivity discrepancies. Next Generation Weather Radar Program, WSR-88D Operational Support Facility, Norman, OK, 25 pp.
- Smith, C.J., 1986: The reduction of errors caused by bright bands in quantitative rainfall measurements made using radar. J. Atmos. Oceanic Tech., 3, 129-141.
- Smith, P.L., 1990: Precipitation measurement and hydrology: Panel report. Radar in Meteorology, D. Atlas Ed., Amer. Meteor. Soc., Boston, 607-618.
- Smith, J.A., and W.F. Krajewski, 1994: Final Report - Estimation of Parameters for the NEXRAD Rainfall Algorithms. NOAA National Weather Service Office of Hydrology - Hydrologic Research Laboratory, Silver Spring, MD.
- ____, D.J. Seo, M.L. Baeck, and M.D. Hudlow, 1996: An intercomparison study of NEXRAD precipitation estimates. Water. Resources Res., 32 (7), 2035-2046.
- Ulbrich, C.W., J.M. Pelissier and L.G. Lee, 1996: Effects of variations in Z-R law parameters and the radar constant on rainfall rates measured by WSR-88D radars. Preprints, 15th Conf. Weather Analysis and Forecasting, Amer. Meteor. Soc., Boston, 316-319.
- Weber, M.E., M.L. Stone, and J.A. Cullen, 1993: Anomalous propagation associated with thunderstorm outflows. Preprints, 26th Conf. Radar Meteor., Amer. Meteor. Soc., Boston, 238-240.
- Wilson, J.W., and E.A. Brandes, 1979: Radar measurement of rainfall. Bull. Amer. Meteor. Soc., 60, 1048-1058.
- Woods, V.S., 1995: A comparison of observed vs WSR-88D estimated rainfall from Tropical Storm Beryl. NWS Southern Region Technical Attachment SR/SSD 95-64, 4 pp. Ft. Worth, TX.
- Zawadzki, I., 1984: Factors affecting the precision of radar measurements of rain. Preprints, 22nd Conf. Radar Meteor., Amer. Meteor. Soc., Boston, 251-256.
BIBLIOGRAPHY
- Steven M. Hunter received the B.S. degree in Meteorology from the University of Wisconsin-Madison in 1979 and the M.S. in Atmospheric Science from the University of Wyoming in 1983. He was employed at NCAR's Convective Storms Division in 1979 and 1980. He served as staff meteorologist in the USAF's Air Weather Service detachment at Vandenberg AFB, CA from 1984-1988. He moved to Norman, OK, where he worked at the National Severe Storms Laboratory (1989-1992) and the WSR-88D Operational Support Facility Hotline (1992-1994). Mr. Hunter's interests are in convective storms, lightning data, radar, cloud physics, hydrometeorology, and the psychology/sociology of weather impacts on the public.
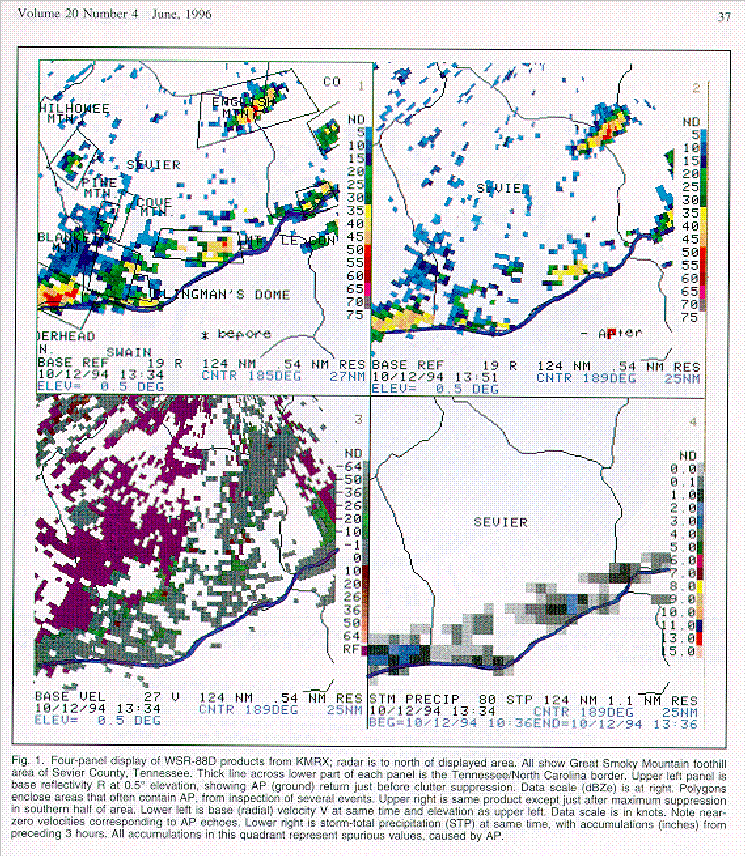
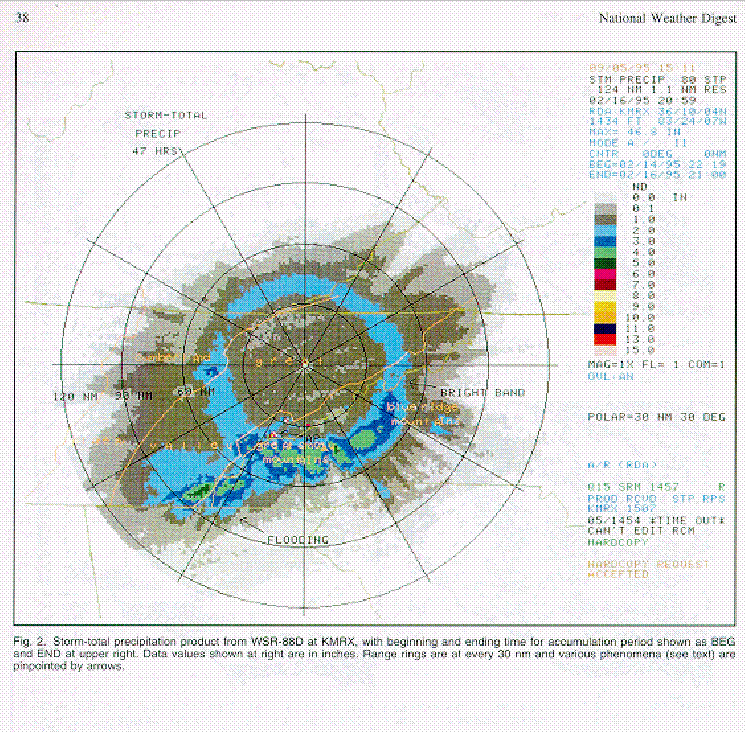
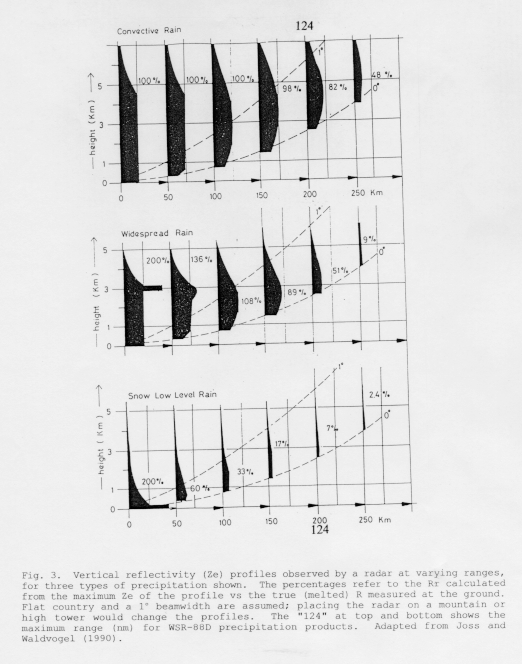
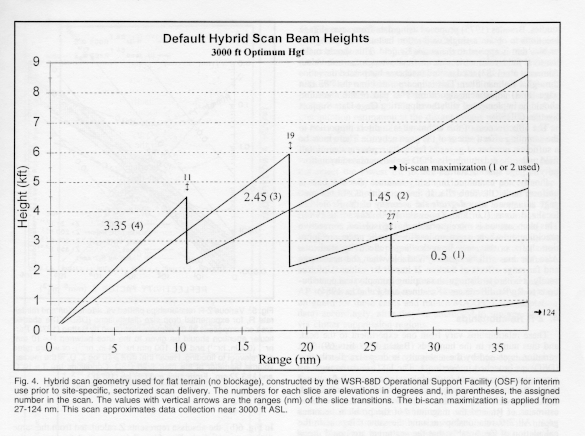
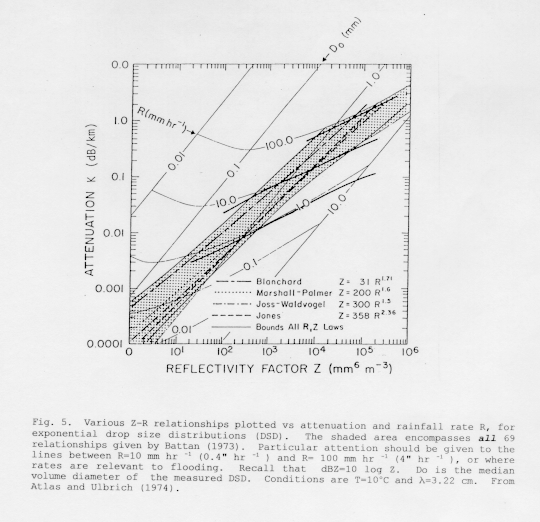
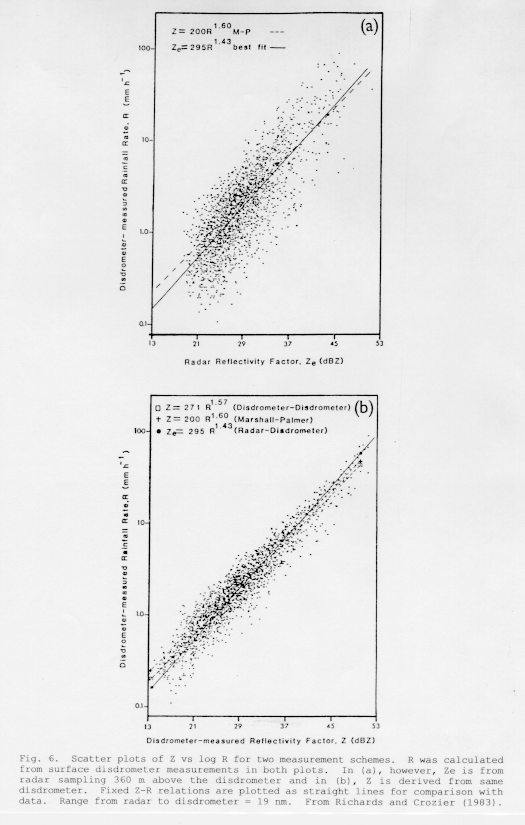
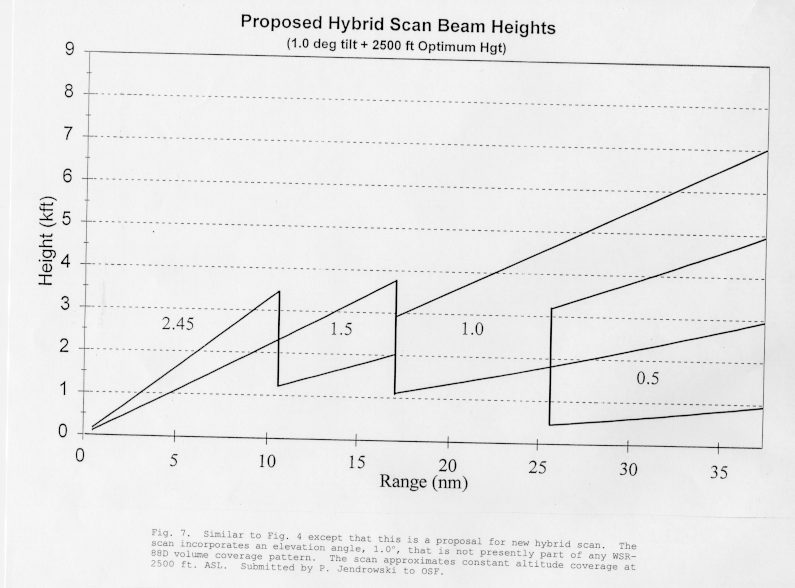